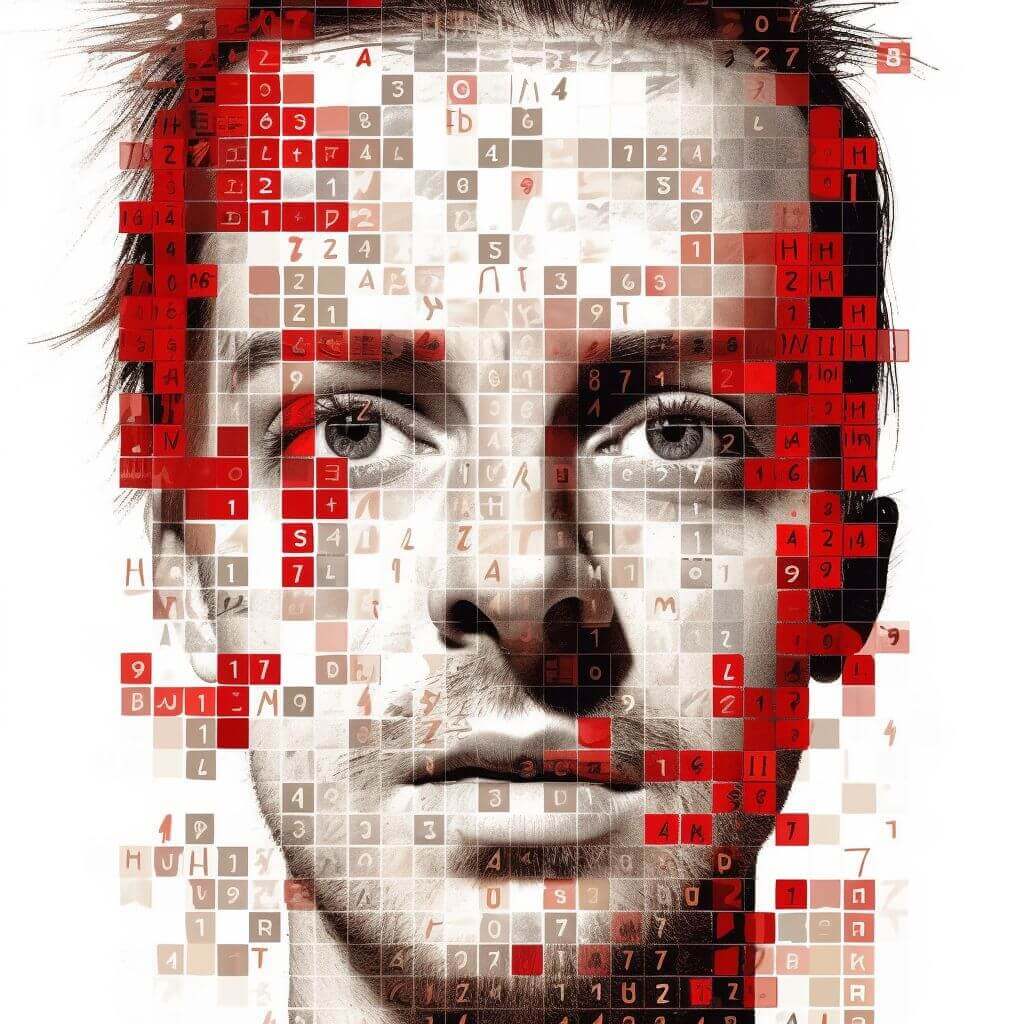
Vector Databases
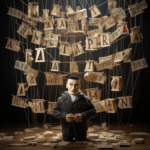
Navigating the Linguistic Labyrinth: The Interplay of AI, Language, and Thought
Blog written by Vaclav Kosar
Follow the post here
How transformers convert text and other data to vectors and back using tokenization, positional encoding, embedding layers.
- Transformer is sequence to sequence neural network architecture
- Input text is encoded with tokenizers to sequence of integers called input tokens
- Input tokens are mapped to sequence of vectors (word embeddings) via embeddings layer
- Output vectors (embeddings) can be classified to a sequence of tokens
- Output tokens can then be decoded back to a text
Tokenization vs Embedding
- Input is tokenized, the tokens then are embedded
- Output text embeddings are classified back into tokens, which then can be decoded into text
- Tokenization converts a text into a list of integers
- Embedding converts the list of integers into a list of vectors (list of embeddings)
- Positional information about each token is added to embeddings using positional encodings or embeddings
Tokenization
- Tokenization is cutting input data into parts (symbols) that can be mapped (embedded) into a vector space.
- For example, input text is split into frequent words e.g. transformer tokenization.
- Sometimes we append special tokens to the sequence e.g. class token ([CLS]) used for classification embedding in BERT transformer.
- Tokens are mapped to vectors (embedded, represented), which are passed into neural neural networks.
- Token sequence position itself is often vectorized and added to the word embeddings (positional encodings).
Positional Encodings add Token Order Information
- Self-attention and feed-forward layers are symmetrical with respect to the input
- So we have to provide positional information about each input token
- So positional encodings or embeddings are added to token embeddings in transformer
- Encodings are manually (human) selected, while embeddings are learned (trained)
Word Embeddings
- Embedding layers map tokens to word vectors (sequence of numbers) called word embeddings.
- Input and output embeddings layer often share the same token-vector mapping.
- Embeddings contain semantic information about the word.
Explore Yourself
Try out BERT BPE tokenizer and its embeddings using Transformers package.
# pip install transformers && pip install torch from transformers import DistilBertTokenizerFast, DistilBertModeltokenizer = DistilBertTokenizerFast.from_pretrained("distilbert-base-uncased") tokens = tokenizer.encode('This is a input.', return_tensors='pt') print("These are tokens!", tokens) for token in tokens[0]: print("This are decoded tokens!", tokenizer.decode([token])) model = DistilBertModel.from_pretrained("distilbert-base-uncased")print(model.embeddings.word_embeddings(tokens)) for e in model.embeddings.word_embeddings(tokens)[0]: print("This is an embedding!", e)